
CorrFlowĭistribution of markup from one image to the entire video.Ĭonverting object images from one class to another with a minimum amount of training data.īased on GAN architecture. The model receives blurred frames at the input of the model, and the restored frames without blur at the output. Restore sharpness when the frame approaches and restore the content of blurry frames in video recording. Some fix for the disadvantages of using GAN for image generation.Ĭommunication system of encoder and decoder on two levels. Generation of realistic versatile images. Increase the precision of marked object boundaries.Īn additional layer to any semantic editor and loss function. STEALĭetection of object boundaries on noisy data. Object recognition from thousands of categories.ĭetection of hard-to-see objects in the image.Īn architecture that allows you to work on top of any existing detector. Solving the instance segmentation problem is 10 times computationally better than other existing approaches.Įnd-to-end neural network that accepts a 3D image as input, and gives out the boundary of recognized objects at the output.
MACHINE LEARNING IMAGE CLEANER SOFTWARE
Ready-made solutions are open-source repositories and software tools that are built to solve particular, often specialized tasks.īy using those solutions you can “outsource” your model building or image processing pipeline to a tool that does it with one(ish) click or one command execution. ImageNet-AĪ dataset of images that the neural network cannot classify correctly.īased on the test results, the models predicted objects from the dataset with an accuracy of 3%.Ĭontains 7.5 thousand images, the peculiarity of which is that they contain natural optical illusions.ĭesigned to study the stability of neural networks to ambiguous images of objects, which will help to increase the generalizing ability of models. Video options include weather, pedestrians, lighting, cyclists, and construction sites.ĭiversity in the data increases the generalization ability of the models that are trained on it. To eliminate the problem of uniformity of videos, the recordings were made under various conditions. Includes videos of driving with marked objects.ģ,000 driving videos totaling 16.7 hours, 600,000 frames, about 25 million 3D object boundaries and 22 million 2D object boundaries.
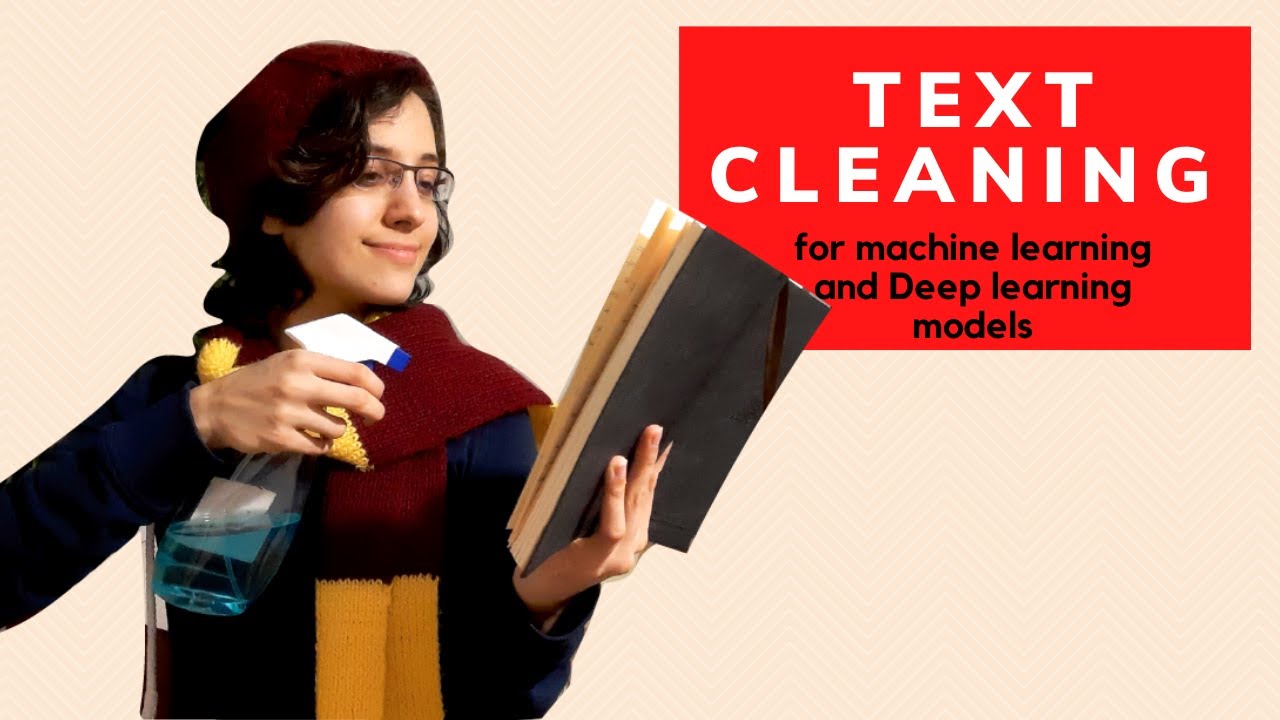
In total, 441,642 objects were marked in the dataset for 31,064 images. The dataset contains directional object boundaries and object classes. Object marking is available for both RGB and infrared images. DroneVehicleĭataset for counting objects in drone images.ġ5,532 RGB drone shots, there is an infrared shot for each image. The data consists of real-world tagged images and unlabeled sketches. SketchTransferĭataset for training neural networks to generalize YouTube-8M Segmentsĭataset of Youtube videos, with marked up content in dynamics.Īpproximately 237 thousand layouts and 1000 categories.

FaceForencisĭataset for recognizing fake photos and videos.Ī set of images (over half a million) created using the Face2Face, FaceSwap and DeepFakes methods.ġ000 videos with faces made using each of the falsification methods. With that in mind, let me give you a list of image datasets that you can use in your projects: Diversity in FacesĪ dataset designed to reduce the bias of algorithms.Ī million labeled images of faces of people of different nationalities, ages and genders, as well as other indicators – head size, face contrast, nose length, forehead height, face proportions, etc. This is especially important in image processing applications where adding more labeled data to your training dataset usually gets you bigger improvements than state-of-the-art network architectures or training methods.

You cannot build machine learning models without the data.
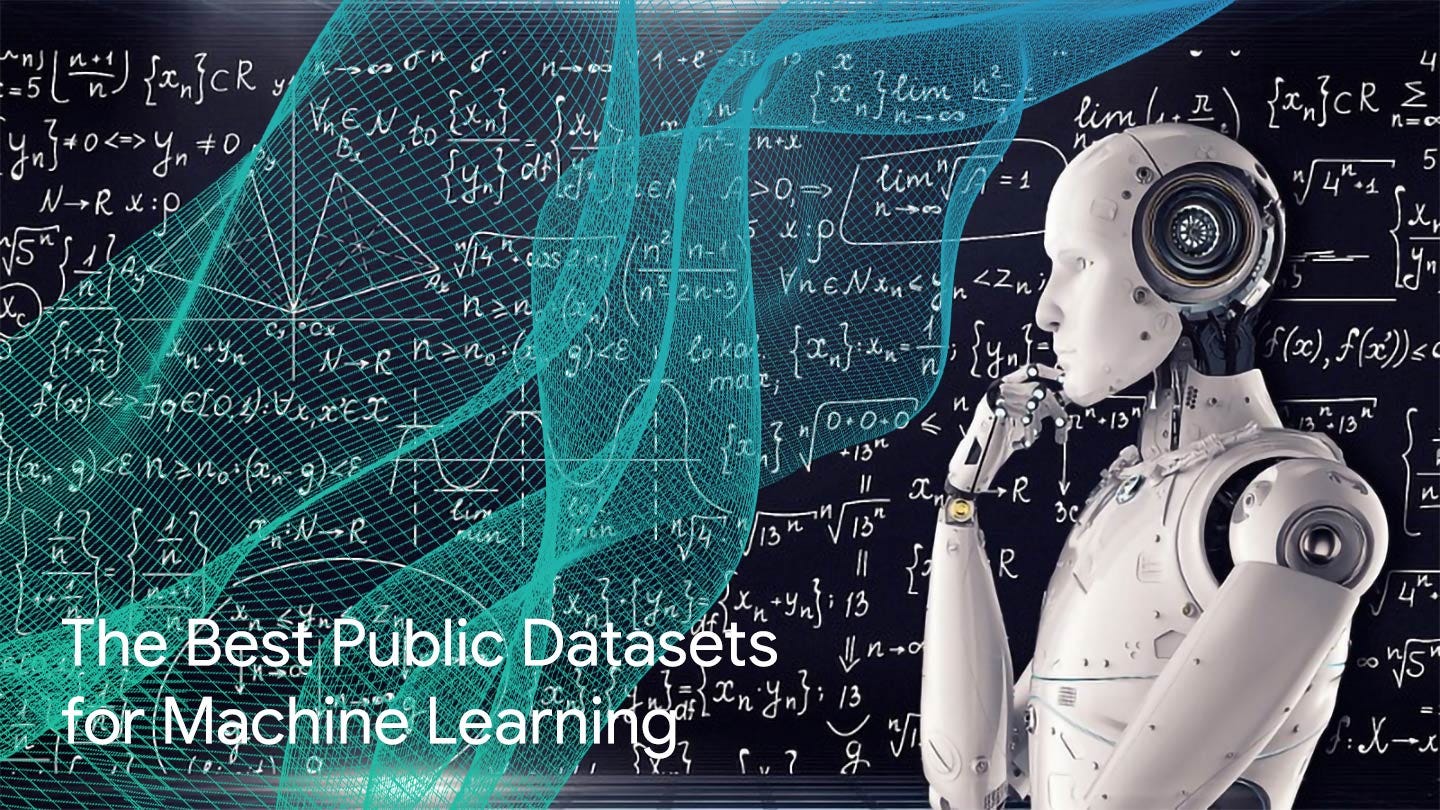
